Welcome to cdp-data’s documentation!¶
cdp-data¶
Data Utilities and Processing Generalized for All CDP Instances
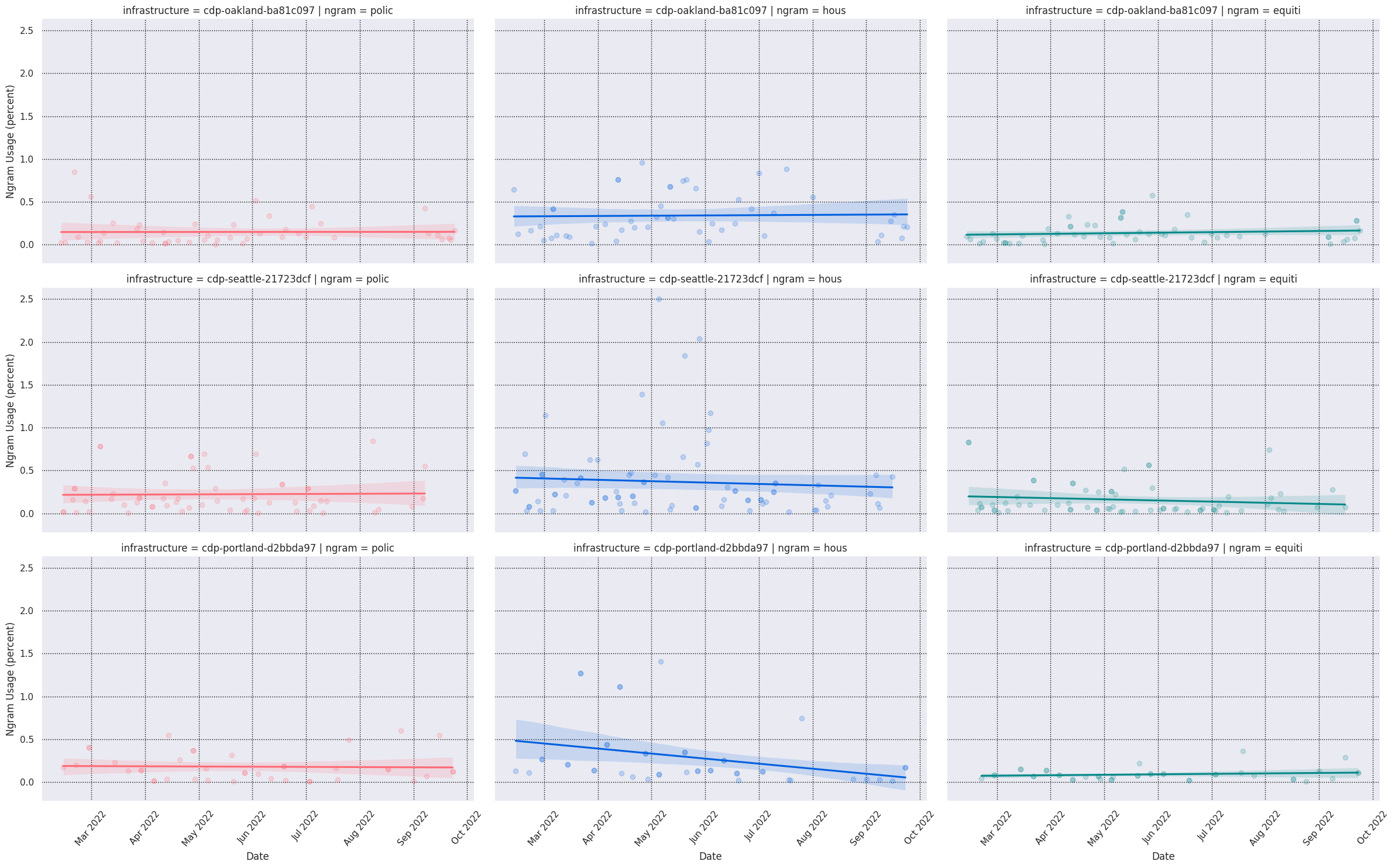
Installation¶
Stable Release: pip install cdp-data
Development Head: pip install git+https://github.com/CouncilDataProject/cdp-data.git
Documentation¶
For full package documentation please visit councildataproject.github.io/cdp-data.
Quickstart¶
Pulling Datasets¶
Install basics: pip install cdp-data
Transcripts and Session Data¶
from cdp_data import CDPInstances, datasets
ds = datasets.get_session_dataset(
infrastructure_slug=CDPInstances.Seattle,
start_datetime="2021-01-01",
store_transcript=True,
)
Transcript Schema and Usage¶
It may be useful to look at our transcript model documentation.
Transcripts can be read into memory and processed as an object:
from cdp_backend.pipeline.transcript_model import Transcript
# Read the file as a Transcript object
with open("transcript.json", "r") as open_f:
transcript = Transcript.from_json(open_f.read())
# Navigate the object
for sentence in transcript.sentences:
if "clerk" in sentence.text.lower():
print(f"{sentence.index}, {sentence.start_time}: '{sentence.text}')
If you do not want to do this processing in Python or prefer to work with a DataFrame, you can convert transcripts to DataFrames like so:
from cdp_data import datasets
# assume that transcript is the same transcript as the prior code snippet
sentences = datasets.convert_transcript_to_dataframe(transcript)
You can also do this conversion (and storage of the coverted transcript) for
all transcripts in a session dataset during dataset construction with the
store_transcript_as_csv
parameter.
from cdp_data import CDPInstances, datasets
ds = datasets.get_session_dataset(
infrastructure_slug=CDPInstances.Seattle,
start_datetime="2021-01-01",
store_transcript=True,
store_transcript_as_csv=True,
)
This will store the transcript for each session as both JSON and CSV.
Voting Data¶
from cdp_data import CDPInstances, datasets
ds = dataset.get_vote_dataset(
infrastructure_slug=CDPInstances.Seattle,
start_datetime="2021-01-01",
)
Data Definitions and Schema¶
Please refer to our database schema and our database model definitions for more information on CDP generated and archived data is structured.
Saving Datasets¶
Because we heavily rely on our database models for database interaction,
in many cases, we default to returning the full fireo.models.Model
object
as column values.
These objects cannot be immediately stored to disk so we provide a helper to replace all model objects with their database IDs for storage.
This can be done directly if you already have a dataset you have been working with:
from cdp_data import datasets
# data should be a pandas dataframe
dataset.save_dataset(data, "data.csv")
Or this can be premptively be done during dataset construction:
from cdp_data import CDPInstances, dataset
# both get_session_dataset and get_vote_dataset
# have a `replace_py_objects` parameter
sessions = datasets.get_session_dataset(
infrastructure_slug=CDPInstances.Seattle,
replace_py_objects=True,
)
votes = datasets.get_vote_dataset(
infrastructure_slug=CDPInstances.Seattle,
replace_py_objects=True,
)
Plotting and Analysis¶
Install plotting support: pip install cdp-data[plot]
Ngram Usage over Time¶
from cdp_data import CDPInstances, keywords, plots
ngram_usage = keywords.compute_ngram_usage_history(
CDPInstances.Seattle,
start_datetime="2022-03-01",
end_datetime="2022-10-01",
)
grid = plots.plot_ngram_usage_histories(
["police", "housing", "transportation"],
ngram_usage,
lmplot_kws=dict( # extra plotting params
col="ngram",
hue="ngram",
scatter_kws={"alpha": 0.2},
aspect=1.6,
),
)
grid.savefig("seattle-keywords-over-time.png")
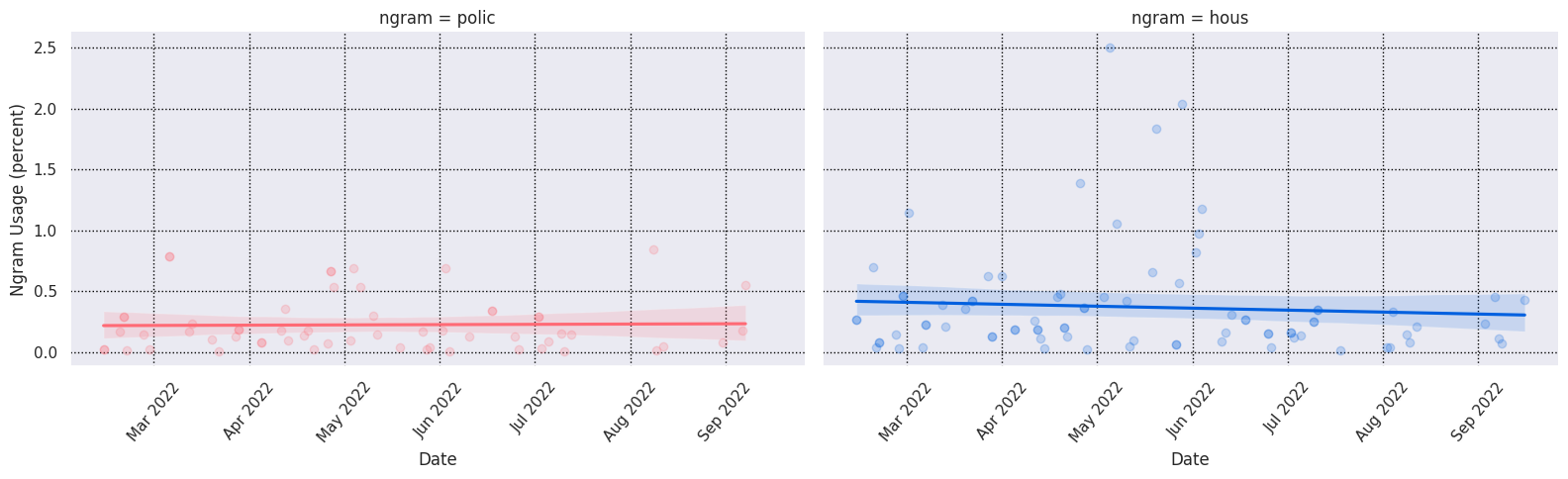
Development¶
See CONTRIBUTING.md for information related to developing the code.
MIT license